Meta-Reinforcement Learning for
Robotic Industrial Insertion Tasks
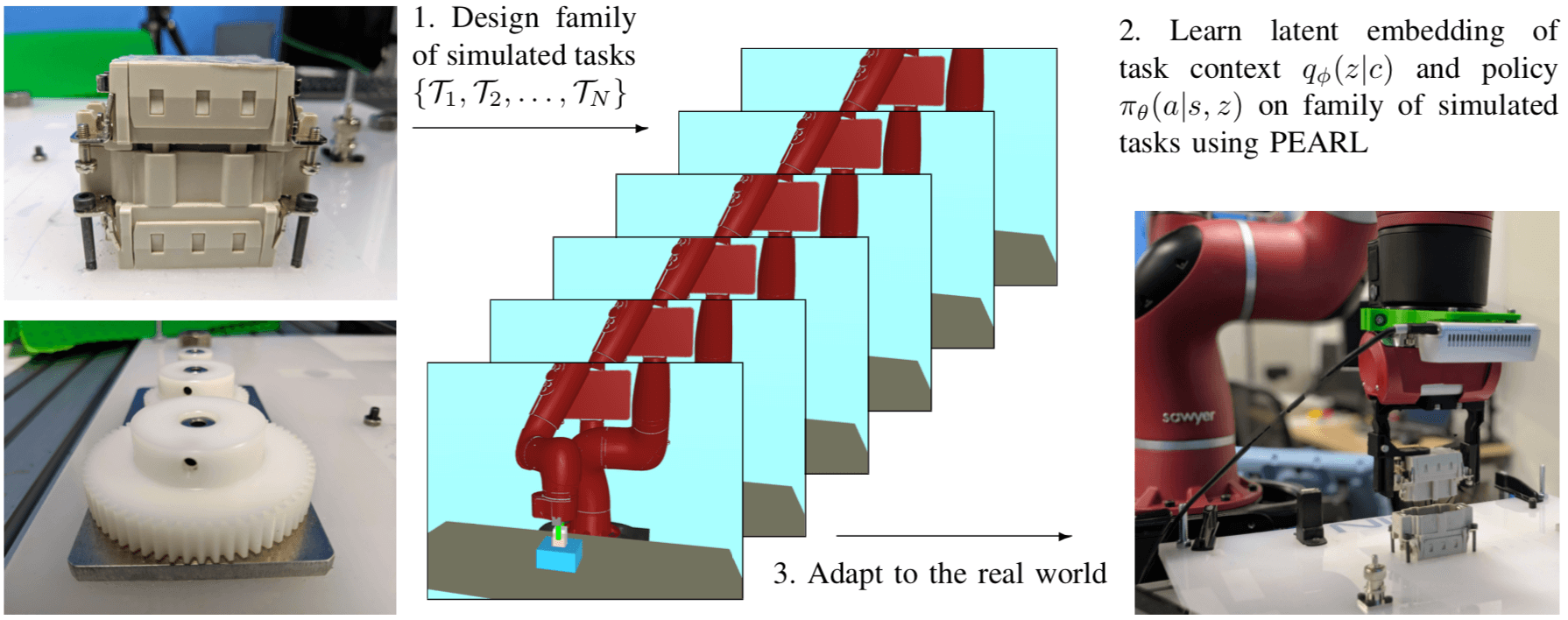
Abstract
Robotic insertion tasks are characterized by contact and friction mechanics, making them challenging for conventional feedback control methods due to unmodeled physical effects. Reinforcement learning (RL) is a promising approach for learning control policies in such settings. However, RL can be unsafe during exploration and might require a large amount of real-world training data, which is expensive to collect. In this paper, we study how to use meta-reinforcement learning to solve the bulk of the problem in simulation by solving a family of simulated industrial insertion tasks and then adapt policies quickly in the real world. We demonstrate our approach by training an agent to successfully perform challenging real-world insertion tasks using less than 20 trials of real-world experience.
Video
Preprint
Preprint can be accessed on arXiv.
Website Template
The template for this website has been adopted from Carl Doersch.
Contact
For comments/questions, contact Ashvin Nair